Generative AI in Healthcare: Opportunities & What's Next
Summary
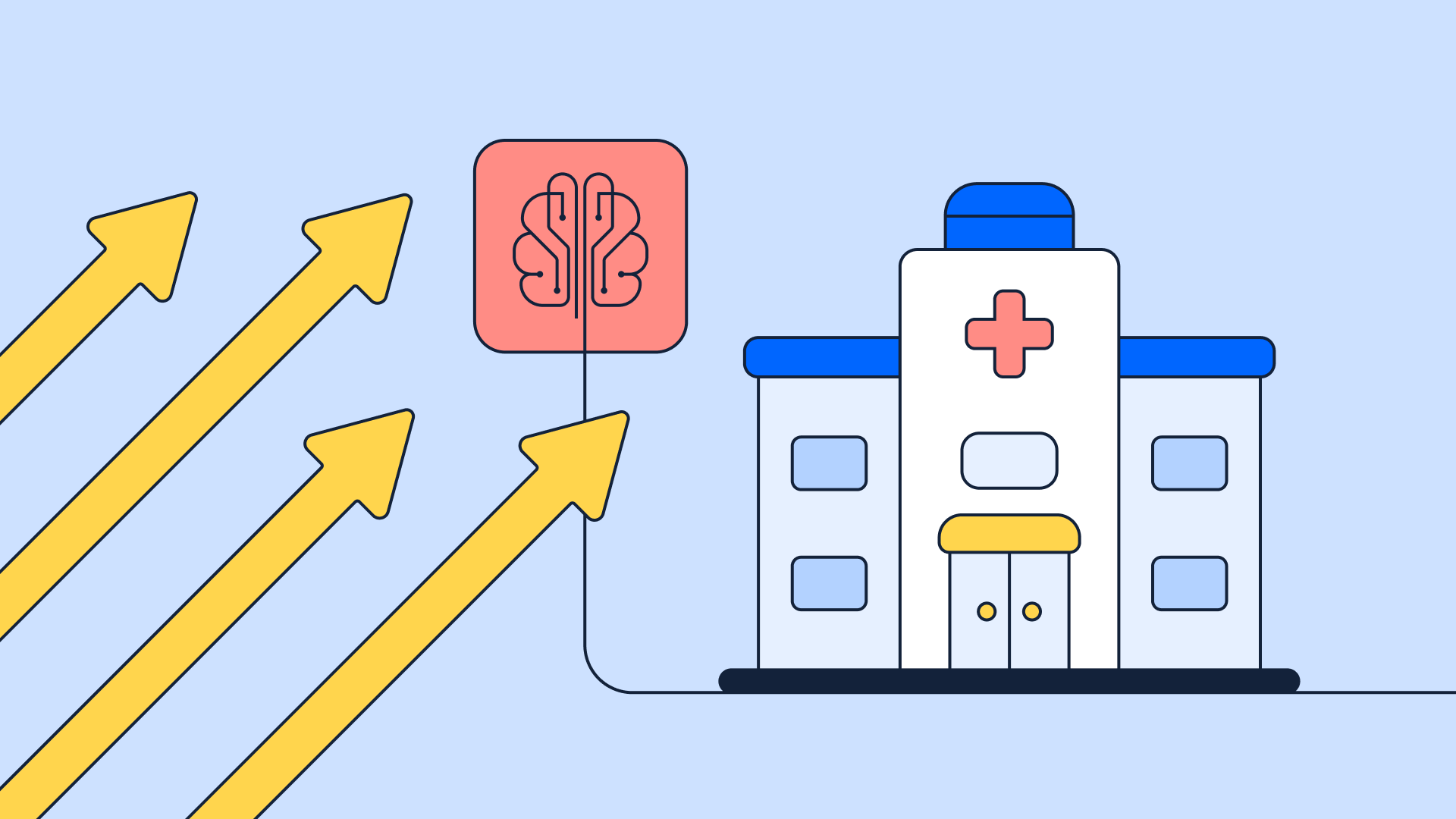
Generative AI in healthcare is poised to revolutionize the industry by automating repetitive tasks and enhancing patient care.
This transformative technology is being utilized to optimize processes like data entry, appointment scheduling, and monitoring vital statistics. This frees healthcare professionals to focus on more complex patient needs.
Generative AI has the potential to significantly accelerate drug development, enhance medical imaging, and improve the efficiency of healthcare systems.
This article explores the potential applications, benefits, and challenges of generative AI in healthcare, shedding light on its impact and future implications.
Generative AI in Healthcare: TL;DR
- AI models enhance the accuracy of medical image interpretation, aiding in the early detection of diseases.
- AI-generated insights tailor patient-specific treatments, optimizing care plans for individual treatments.
- AI accelerates the discovery of new drugs, paving the way for innovative new therapies.
- AI automates routine tasks, freeing healthcare professionals to focus on direct patient care.
What Is Generative AI and How Does It Work?
Generative AI is a branch of artificial intelligence focused on creating new content. This technology utilizes machine learning models to produce text, images, audio, and video. By learning from large datasets, these models can deliver content that mimics human-like creativity.
The core of generative AI lies in advanced deep-learning algorithms. These algorithms are designed to recognize patterns in data, enabling the generation of novel outputs. A common technique used is Generative Adversarial Networks (GANs).
GANs consist of two main components: a generator and a discriminator. The generator creates data, while the discriminator evaluates it against real-world examples. This adversarial process helps refine the generator's output.
Another popular tool is the transformer model, responsible for many recent advancements. Transformers use self-attention mechanisms to process sequences of data, offering impressive language understanding capabilities.
Generative AI has numerous applications in healthcare. It can automate routine tasks, such as data entry, and support patient care by monitoring vital signs. Its ability to process vast amounts of medical data allows for efficient document review and data extraction.
Despite its potential, generative AI also presents challenges. These include ethical considerations regarding data privacy and the quality of generated content.
Ongoing research aims to address these concerns, ensuring the safe and effective use of this technology in healthcare settings.
5 Benefits of Gen AI in Healthcare
Generative AI in healthcare is revolutionizing the sector, bringing with it a myriad of benefits to patients, healthcare professionals, and administrative staff. Here are the top five benefits of combining generative AI and healthcare.
1. Enhanced Clinical Decision-Making
Gen AI assists healthcare providers by offering evidence-based recommendations. By analyzing vast amounts of data, including diagnostic reports and medical literature, it supports clinicians in making more informed decisions.
2. Streamlined Administrative Tasks
Gen AI use cases in healthcare show that automating routine administrative processes such as scheduling, billing, and record management reduces the time healthcare professionals spend on these duties, allowing them to focus more on patient care.
3. Personalized Medicine
Gen AI enables the development of personalized treatment plans. By evaluating individual genetic information along with medical histories, it tailors interventions to suit each patient’s unique needs, potentially improving outcomes.
4. Drug Discovery Acceleration
The pharmaceutical industry benefits from Gen AI's efficient identification of potential drug candidates. By simulating biological interactions, it speeds up the initial phases of drug development, leading to quicker clinical trials and potential therapies.
5. Patient Engagement and Adherence
AI systems engage patients by providing timely reminders and education about their conditions. This promotes adherence to treatment plans and fosters proactive patient involvement, ultimately enhancing overall health outcomes.
6 Applications of Generative AI in Healthcare
Generative AI has the potential to revolutionize healthcare through various applications. It enhances operational efficiency and accelerates medical research. Here are six generative AI healthcare use cases.
1. Drug Discovery
Generative AI assists researchers in identifying new drug candidates by analyzing vast datasets. It speeds up the drug development process, allowing for more effective treatments.
2. Clinical Documentation
It automates the creation of medical records, reducing the administrative burden on healthcare professionals. This leads to more accurate documentation and frees up time for patient care.
3. Medical Imaging
AI models enhance medical imaging interpretation by identifying patterns and anomalies in scans. This improves diagnostic accuracy and supports early detection of diseases.
4. Clinical Trials
Generative AI optimizes the design and execution of clinical trials. It identifies potential candidate populations and predicts their responses, making trials more efficient.
5. Personalized Medicine
AI technologies analyze genetic data to offer tailored treatment plans. This personalization ensures patients receive the most suitable therapies based on their individual profiles.
6. Predictive Maintenance In Healthcare Facilities
AI predicts equipment failures and schedules maintenance. This minimizes downtime and ensures the availability of critical medical devices.
Best Practices for Effective Gen AI Implementation in Healthcare
Integrate AI Into Existing Structures
To integrate generative AI into healthcare, organizations should infuse AI into their existing structures. This involves training staff on the potential benefits and limitations of AI technologies, ensuring everyone is well-prepared for AI-driven transformations.
Establish an AI Operational Foundation
Establishing an AI Operational Foundation is essential. Health institutions should facilitate cross-disciplinary collaboration between IT professionals, clinicians, and administrative staff to streamline AI processes and foster an AI-friendly culture.
Develop a Robust AI Infrastructure Plan
Developing a robust AI Infrastructure Plan is crucial for managing data effectively. This includes securing high-quality datasets, prioritizing data privacy, and setting up cloud-based solutions where necessary. Institutions should assess their existing data frameworks and consider upgrades for efficient AI deployment.
Start with Pilot Programs
AI initiatives should begin with experimentation and pilot programs. Identifying high-value, low-risk AI applications aids in establishing trust and overcoming initial implementation hurdles. These programs allow for practical assessments and refinement of AI systems before broader application.
Prioritize Security and Compliance
Security and compliance are non-negotiable. Ensuring AI systems adhere to healthcare regulations, such as HIPAA, protects sensitive patient information. Regular audits and updates to safeguard data integrity are indispensable.
Align AI with Clinical Goals
Finally, aligning AI solutions with clinical goals ensures they contribute effectively to healthcare improvements. By focusing on enhancing clinical decision-making and patient outcomes, AI can be a transforming tool in medical settings.
Embrace Continuous Improvement
Adjusting strategies based on continuous feedback and measurable outcomes is important to ensure ongoing success.
Generative AI for Healthcare: Future Trends
Generative AI is significantly influencing the future of healthcare by improving efficiency and innovation. Numerous healthcare organizations are planning to integrate AI solutions across their operations. This technology is particularly valued for its potential to streamline processes and enhance decision-making. Let’s take a look at some future generative AI in healthcare examples.
Patient Care and Experience
AI-driven tools are being developed to offer personalized patient communications. These tools aim to improve engagement and adherence to treatment plans, resulting in better outcomes. Moreover, AI is seen as key in tailoring treatment plans based on a patient's unique data.
Drug Discovery and Clinical Trials
Generative AI is accelerating drug discovery by identifying potential compounds and optimizing their properties. The technology is also vital in designing and managing clinical trials, making them more efficient and less costly.
Administrative Efficiency
Healthcare systems are looking to generative AI to reduce administrative burdens. By automating routine tasks such as scheduling and billing, AI frees up healthcare professionals to focus more on patient care.
Medical Imaging and Diagnostics
AI tools are rapidly advancing in their ability to improve medical imaging. Organizations are investing in AI applications that assist in early and accurate diagnosis. These tools enhance radiologists' ability to interpret scans, ultimately leading to better diagnosis and treatment.
Governance and Ethics
As generative AI becomes more prevalent, companies are implementing governance and oversight structures. These are essential to ensure ethical use and compliance with legal requirements. Monitoring AI performance and addressing biases are central to maintaining trust and safety in healthcare applications.
Final Thoughts on Generative AI in Healthcare
Generative AI is not just a futuristic concept—it's already reshaping the landscape of healthcare today. From accelerating drug discovery and enhancing diagnostic accuracy to streamlining administrative workflows and personalizing patient care, its potential is both vast and transformative.
However, realizing this potential requires more than just adopting new technology. It demands strategic integration, a strong ethical framework, and continuous evaluation to ensure effectiveness and safety.
As healthcare organizations embrace generative AI, those that prioritize clinical alignment, compliance, and ongoing innovation will be best positioned to lead the charge into a smarter, more efficient future of medicine.
FAQs
What are the main challenges in using generative AI in medicine?
One of the primary challenges is ensuring data privacy and security. Medical records contain sensitive information, and any AI system must comply with strict data protection regulations, such as the Health Insurance Portability and Accountability Act (HIPAA) in the United States.
Another issue is the accuracy and reliability of AI-generated outputs. While generative AI can assist in diagnosis and treatment planning, the algorithms can sometimes produce incorrect predictions. This necessitates the use of robust validation methods before deploying such technologies clinically.
Moreover, there is a need for integration with existing healthcare systems to ensure seamless operation without disrupting existing workflows. Training healthcare professionals to effectively use these technologies is also critical to overcoming adoption barriers.
Are there any legal regulations regarding the use of generative AI in healthcare?
Yes, numerous regulations govern the use of generative AI in healthcare. In the United States, the Food and Drug Administration (FDA) plays a key role in approving medical AI applications. It's crucial for AI tools used in diagnosis or treatment to comply with FDA standards to ensure safety and efficacy.
The General Data Protection Regulation (GDPR) in Europe imposes strict guidelines on personal data handling, affecting how AI systems process patient data. Compliance not only involves technical measures but also administrative practices to ensure ethical use.
These regulations are continually evolving, so staying informed about legal changes is essential for developers and healthcare providers alike.
How big is the gen AI market in healthcare?
The generative AI market in healthcare is expanding rapidly. Industry reports estimate the market value could reach several billion dollars within the next few years. This growth is driven by increasing investments in AI start-ups and enhanced applications in fields like radiology, oncology, and personalized medicine.
Factors contributing to this expansion include technological advancements, increased data availability, and rising demand for innovative healthcare solutions. The market's rapid development highlights the strategic importance of generative AI in improving healthcare delivery and outcomes.
Which is the best AI in the healthcare sector?
Determining the best AI in healthcare is challenging due to the diverse range of applications and functionalities.
Notable AI platforms include IBM Watson Health, which specializes in oncology and genomics, and Google's DeepMind, known for its work in neural networks and predictive analytics.
Other significant players, such as Microsoft’s Azure Healthcare and AWS Machine Learning, offer robust cloud-based solutions tailored for healthcare needs.
Each has its strengths, often depending on the specific use case, such as patient management, imaging, or data analysis.
Decision-makers should assess AI tools based on their specific healthcare needs and compatibility with existing systems.